Comparison of Spectral and Sparse Feature Extraction Methods for Heart Sounds Classification
DOI:
https://doi.org/10.17488/RMIB.44.4.1Keywords:
classification, heart sounds, matching pursuit, spectral features, time-frequency representationAbstract
Cardiovascular diseases (CVDs) remain the leading cause of morbidity worldwide. The heart sound signal or phonocardiogram (PCG) is the most simple, low-cost, and effective tool to assist physicians in diagnosing CVDs. Advances in signal processing and machine learning have motivated the design of computer-aided systems for heart illness detection based only on the PCG. The objective of this work is to compare the effects of using spectral and sparse features for a classification scheme to detect the presence/absence of a pathological state in a heart sound signal, more specifically, sparse representations using Matching Pursuit with multiscale Gabor time-frequency dictionaries, linear prediction coding, and Mel-frequency cepstral coefficients. This work compares the performance of PCGs classification applying features as a result of averaging the samples or the features for each PCG sound event when feeding a random forest (RF) classifier. For data balancing, random under-sampling and synthetic minority oversampling (SMOTE) methods were applied. Furthermore, we compare the Correlation Feature Selection (CFS) and Information Gain (IG) for the dimensionality reduction. The findings show a SE=93.17 %, SP=84.32 % and ACC=85.9 % when joining MP+LPC+MFCC features set with an AUC=0.969 showing that these features are promising to be used in heart sounds anomaly detection schemes.
Downloads
References
World Health Organization (WHO), “Cardiovascular diseases (CVDs),” WHO. Available: http://www.who.int/mediacentre/factsheets/fs317/en/ (accessed 2022).
A. K. Abbas, R. Bassam, “Phonocardiography signal processing,” Springer Cham, 2009, pp. 194. [Online]. Available: https://doi.org/10.2200/S00187ED1V01Y200904BME031
G. D. Clifford, C. Liu, B. Moody, D. Springer, I. Silva, Q. Li, R. G. Mark, “Classification of normal/abnormal heart sound recordings: The physionet/computing in cardiology challenge 2016”, in: 2016 Computing in Cardiology Conference (CinC), Vancouver, BC, Canada, 2016, pp. 609-612. [Online]. Available: https://ieeexplore.ieee.org/document/7868816
W. Zhang, J. Han, S. Deng, “Abnormal heart sound detection using temporal quasi-periodic features and long short-term memory without segmentation,” Biomed. Signal Process. Control, vol. 53, art. no. 101560, Aug. 2019, doi: https://doi.org/10.1016/j.bspc.2019.101560
Y. Soeta, Y. Bito, Y. “Detection of features of prosthetic cardiac valve sound by spectrogram analysis,” Appl. Acoust., vol. 89, pp. 28-33, Mar. 2015, doi: https://doi.org/10.1016/j.apacoust.2014.09.003
L. Orozco-Reyes, M.-Á. A. Arévalo, E. García-Canseco, R. F. Ibarra-Hernández, “Clasificación de la senal de audio cardiaco mediante la transformada de Fourier de tiempo corto y aprendizaje profundo,” Res. Comput. Sci., vol. 151, no. 7, pp. 141-155, 2022. [Online]. Available: http://148.204.65.169/2022_151_7/Clasificacion%20de%20la%20senal%20de%20audio%20cardiaco%20mediante%20la%20transformada%20de%20Fourier%20de%20tiempo%20corto%20y.pdf
M. S. Obaidat, “Phonocardiogram signal analysis: techniques and performance comparison,” J. Med. Eng. Technol., vol. 17 no. 6, pp. 221-227, 1993, doi: https://doi.org/10.3109/03091909309006329
N. E., Huang, Z. Shen, S. R. Long, M. C. Wu, H. H. Shih, Q. Zheng, N.-C. Ten, C. C. Tung, H. H. Liu, “The empirical mode decomposition and the Hilbert spectrum for nonlinear and non-stationary time series analysis,” Proc. R. Soc. Lond., vol. 454, no. 1971, pp. 903-995, Mar. 1998, doi: https://doi.org/10.1098/rspa.1998.0193
V. Nivitha Varghees, K. I. Ramachandran, “Effective heart sound segmentation and murmur classification using empirical Wavelet transform and instantaneous phase for electronic stethoscope,” IEEE Sens. J., vol. 17, no. 12, pp. 3861–3872, Jun. 2017, doi: https://doi.org/10.1109/JSEN.2017.2694970
S. Yuenyong, A. Nishihara, W. Kongprawechnon, K. Tungpimolrut, “A framework for automatic heart sound analysis without segmentation,” BioMed. Eng. OnLine, no. 10, art. no. 13, Feb. 2011, doi: https://doi.org/10.1186/1475-925X-10-13
S. M. Debbal, F. Bereksi-Reguig, “Detection of Differences of the Phonocardiogram Signals by Using the Continuous Wavelet Transform Method,” Int. J. Biomedical Soft Computing Hum. Sci., vol. 18 no.2, pp. 73-81, 2013, doi: https://doi.org/10.24466/ijbschs.18.2_73
B. Ergen, Y. Tatar, H. O. Gulcur, “Time–frequency analysis of phonocardiogram signals using Wavelet transform: a comparative study,” Comput. Methods Biomech. Biomed. Eng., vol. 15, no. 4, pp. 371-381, Jan. 2011 doi: https://doi.org/10.1080/10255842.2010.538386
S. A. Singh, T. G. Meitei, S. Majumder, “Short PCG classification based on deep learning,” in Deep Learning Techniques for Biomedical and Health Informatics, B. Agarwal, V. E. Balas, L. C. Jain, R. C. Poonia, Manisha, Eds. London, United Kingdom: Academic Press, 2020, ch. 6, pp. 141–164. [Online]. Doi: https://doi.org/10.1016/B978-0-12-819061-6.00006-9
P. Qiao, Z. Yu, Z. Jingyi, C. Zhuo, “A method for diagnosing heart sounds in adolescents based on wavelet analysis and random forest,” in 2020 International Conference on Big Data, Artificial Intelligence and Internet of Things Engineering (ICBAIE), Fuzhou, China, 2020, pp. 69-74, doi: https://doi.org/10.1109/ICBAIE49996.2020.00021
M. Abdollahpur, S. Ghiasi, M. J. Mollakazemi, A. Ghaffari, “Cycle selection and neuro-voting system for classifying heart sound recordings,” in 2016 Computing in Cardiology Conference (CinC), Vancouver, BC, Canada, 2016, pp. 1-4. [Online] Available: https://ieeexplore.ieee.org/document/7868814
P. Lubaib, K.V. Ahammed Muneer, “The Heart Defect Analysis Based on PCG Signals Using Pattern Recognition Techniques,” Proc. Technol., vol. 24, pp. 1024-1031, 2016, doi: https://doi.org/10.1016/j.protcy.2016.05.225
M. Zabihi, A. B. Rad, S. Kiranyaz, M. Gabbouj, A. K. Katsaggelos, Heart sound anomaly and quality detection using ensemble of neural networks without segmentation, in 2016 Computing in Cardiology Conference (CinC), Vancouver, BC, Canada, 2016, pp. 613-616. [Online]. Available: https://ieeexplore.ieee.org/document/7868817
P. Wang, C. S. Lim, S. Chauhan, J. Y. A. Foo, V. Anantharaman, “Phonocardiographic signal analysis method using a modified hidden Markov model,” Ann. Biomed. Eng., vol. 35, pp. 367-374, 2007, doi: https://doi.org/10.1007/s10439-006-9232-3
Y. Zheng, X. Guo, X. Ding, “A novel hybrid energy fraction and entropy-based approach for systolic heart murmurs identification,” Expert Syst. Appl., vol. 42, no. 5, pp. 2710-2721, Apr. 2015, doi: https://doi.org/10.1016/j.eswa.2014.10.051
S. Chauhan, P. Wang, C. Sing Lim, V. Anantharaman, “A computer-aided MFCC-based HMM system for automatic auscultation,” Comput. Biol. Med., vol. 38, no. 2, pp. 221-233, Feb. 2008, doi: https://doi.org/10.1016/j.compbiomed.2007.10.006
V. Maknickas, A. Maknickas, “Recognition of normal–abnormal phonocardiographic signals using deep convolutional neural networks and mel-frequency spectral coefficients,” Physiol. Meas., vol. 38, no. 8, art. no. 1671, Jul. 2017, doi: https://doi.org/10.1088/1361-6579/aa7841
G. Redlarski, D. Gradolewski, A. Palkowski, “A System for Heart Sounds Classification,” PLoS One, vol. 9, no. 11, art. no. e112673, Nov. 2014, doi: https://doi.org/10.1371/journal.pone.0112673
B. M. Whitaker, P. B. Suresha, C. Liu, G. D. Clifford, D. V. Anderson, “Combining sparse coding and time-domain features for heart sound classification,” Physiol. Meas., vol. 38, no. 8, art. no. 1701, Jul. 2017, doi: https://doi.org/10.1088/1361-6579/aa7623
I. J. Diaz Bobillo, “A tensor approach to heart sound classification,” in 2016 Computing in Cardiology Conference (CinC), Vancouver, BC, Canada, 2016, pp. 629–632. [Online]. Available: https://ieeexplore.ieee.org/document/7868821
W. Zhang, J. Han, S. Deng, “Heart sound classification based on scaled spectrogram and tensor decomposition,” Expert Syst. Appl., vol. 84, pp. 220-231, Oct. 2017, doi: https://doi.org/10.1016/j.eswa.2017.05.014
W. Zhang, J. Han, S. Deng, “Heart sound classification based on scaled spectrogram and partial least squares regression,” Biomed. Signal Process. Control, vol. 32, pp. 20-28, Feb. 2017, doi: https://doi.org/10.1016/j.bspc.2016.10.004
P. Banerjee, A. Mondal, “An Irregularity Measurement Based Cardiac Status Recognition Using Support Vector Machine,” J. Med. Eng., vol. 2015, art. no. 327534, Oct. 2015 doi: http://dx.doi.org/10.1155/2015/327534
Y. Zheng, X. Guo, J. Qin, S. Xiao, “Computer-assisted diagnosis for chronic heart failure by the analysis of their cardiac reserve and heart sound characteristics,” Comput. Methods Programs Biomed., vol. 122, no. 3, pp. 372-383, Dec. 2015, doi: https://doi.org/10.1016/j.cmpb.2015.09.001
I. Maglogiannis, E. Loukis, E. Zafiropoulos, A. Stasis, “Support vectors machine-based identification of heart valve diseases using heart sounds,” Comput. Methods Programs Biomed., vol. 95, no. 1, pp. 47-61, Jul. 2009, doi: https://doi.org/10.1016/j.cmpb.2009.01.003
A. F. Quiceno-Manrique, J. I. Godino-Llorente, M. Blanco-Velasco, G. Castellanos-Dominguez, “Selection of Dynamic Features Based on Time–Frequency Representations for Heart Murmur Detection from Phonocardiographic Signals,” Ann. Biomed. Eng., vol. 38, pp. 118-137, 2010, doi: https://doi.org/10.1007/s10439-009-9838-3
L. D. Avendaño-Valencia, J. I. Godino-Llorente, M. Blanco-Velasco, G. Castellanos-Dominguez, “Feature Extraction From Parametric Time–Frequency Representations for Heart Murmur Detection,” Ann. Biomed. Eng., vol. 38, pp. 2716-2732, Jun. 2010, doi: https://doi.org/10.1007/s10439-010-0077-4
Singh, S. A., & Majumder, S. (2019). Classification of unsegmented heart sound recording using KNN classifier,” J. Mech. Med. Biol., vol. 19, no. 04, art. no. 1950025, doi: https://doi.org/10.1142/S0219519419500258
A. Sofwan, I. Santoso, H. Pradipta, M. Arfan A. A. Zahra M, “Normal and Murmur Heart Sound Classification Using Linear Predictive Coding and k-Nearest Neighbor Methods,” in 2019 3rd International Conference on Informatics and Computational Sciences (ICICoS), Semarang, Indonesia, 2019, pp. 1-5, doi: https://doi.org/10.1109/ICICoS48119.2019.8982393
P. Narváez, S. Gutierrez, W. S. Percybrooks, “Automatic Segmentation and Classification of Heart Sounds Using Modified Empirical Wavelet Transform and Power Features,” Appl. Sci., vol. 10 no.14, art. no. 4791, doi: https://doi.org/10.3390/app10144791
M. N. Homsi, N. Medina, M. Hernandez, N. Quintero, G. Perpiñan, A. Quintana, P. Warrick, “Automatic heart sound recording classification using a nested set of ensemble algorithms,” in 2016 Computing in Cardiology Conference (CinC), Vancouver, BC, Canada, 2016, pp. 817-820. [Online]. Available: https://ieeexplore.ieee.org/document/7868868
C. H. Antink, J. Becker, S. Leonhardt, M. Walter, “Nonnegative matrix factorization and random forest for classification of heart sound recordings in the spectral domain,” in 2016 Computing in Cardiology Conference (CinC), Vancouver, BC, Canada, 2016, pp. 809-812. [Online]. Available: https://ieeexplore.ieee.org/document/7868866
N. E. Singh-Miller, N. Singh-Miller, “Using spectral acoustic features to identify abnormal heart sounds,” in 2016 Computing in Cardiology Conference (CinC), Vancouver, BC, Canada, 2016, pp. 557-560. [Online]. Available: https://ieeexplore.ieee.org/document/7868803
C. Potes, S. Parvaneh, A. Rahman, B. Conroy, “Ensemble of feature-based and deep learning-based classifiers for detection of abnormal heart sounds,” in 2016 Computing in Cardiology Conference (CinC), Vancouver, BC, Canada, 2016, pp. 621–624. [Online]. Available: https://ieeexplore.ieee.org/document/7868819
T.-E. Chen, S.-I Yang, L.-T. Ho, K.-H. Tsai, Y.-H. Chen, Y.-F. Chang, Y.-H. Lai, S.-S. Wang, Y. Tsao, C.-C. Wu, “S1 and S2 Heart Sound Recognition Using Deep Neural Networks,” IEEE Trans. Biomed. Eng., vol. 64, no. 2, pp. 372-380, Feb. 2017, doi: https://doi.org/10.1109/TBME.2016.2559800
F. Beritelli, G. Capizzi, G. Lo Sciuto, C. Napoli, F. Scaglione, “Automatic heart activity diagnosis based on Gram polynomials and probabilistic neural networks,” Biomed. Eng. Lett., no. 8, pp. 77-85, Feb. 2018, doi: https://doi.org/10.1007/s13534-017-0046-z
F. Li, H. Tang, S. Shang, K. Mathiak, F. Cong, “Classification of Heart Sounds Using Convolutional Neural Network,” Appl. Sci., vol. 10, no. 11, art. no. 3956, Jun. 2020, doi: https://doi.org/10.3390/app10113956
F. Demir, A. Şengür, V. Bajaj, K. Polat, “Towards the classification of heart sounds based on convolutional deep neural network,” Health Inf. Sci. Syst., vol. 7, art. no. 16, Aug. 2019, doi: https://doi.org/10.1007/s13755-019-0078-0
Y. Chen, S. Wei, Y. Zhang, “Classification of heart sounds based on the combination of the modified frequency wavelet transform and convolutional neural network,” Med. Biol. Eng. Comput., vol. 58, pp. 2039-2047, Jul. 2020, doi: https://doi.org/10.1007/s11517-020-02218-5
F. Renna, J. Oliveira, M. T. Coimbra, “Deep Convolutional Neural Networks for Heart Sound Segmentation,” IEEE J. Biomed. Health Inform., vol. 23, no. 6, pp. 2435-2445, Nov. 2019, doi: https://doi.org/10.1109/JBHI.2019.2894222
F. Li, M. Liu, Y. Zhao, L. Kong, L. Dong, X. Liu, M. Hui, “Feature extraction and classification of heart sound using 1D convolutional neural networks,” EURASIP J. Adv. Signal Process., vol. 2019, art. no. 59, Dec. 2019, doi: https://doi.org/10.1186/s13634-019-0651-3
B. Xiao, Y. Xu, X. Bi, J. Zhang, X. Ma, “Heart sounds classification using a novel 1-D convolutional neural network with extremely low parameter consumption,” Neurocomputing, vol. 392, pp. 153-159, Jun. 2020, doi: https://doi.org/10.1016/j.neucom.2018.09.101
S. Latif, M. Usman, R. Rana, J. Qadir, “Phonocardiographic Sensing Using Deep Learning for Abnormal Heartbeat Detection,” IEEE Sens. J., vol. 18, no. 22, pp. 9393-9400, Nov. 2018, doi: https://doi.org/10.1109/JSEN.2018.2870759
F. A. Khan, A. Abid, M. S. Khan, “Automatic heart sound classification from segmented/unsegmented phonocardiogram signals using time and frequency features,” Physiol. Meas., vol. 41, no. 5, art. no. 055006, Jun. 2020, doi: https://doi.org/10.1088/1361-6579/ab8770
A. Raza, A. Mehmood, S. Ullah, M. Ahmad, G. S. Choi, B.-W. On, “Heartbeat Sound Signal Classification Using Deep Learning,” Sensors, vol. 19, no. 21, art. no. 4819, Nov. 2019, doi: https://doi.org/10.3390/s19214819
E. Messner, M. Zöhrer, F. Pernkopf, “Heart Sound Segmentation—An Event Detection Approach Using Deep Recurrent Neural Networks,” IEEE Trans. Biomed. Eng., vol. 65, no. 9, pp. 1964-1974, Sep. 2018, doi: https://doi.org/10.1109/TBME.2018.2843258
A. K. Dwivedi, S. A. Imtiaz, E. Rodriguez-Villegas, “Algorithms for Automatic Analysis and Classification of Heart Sounds–A Systematic Review,” IEEE Access, vol. 7, pp. 8316-8345, 2019, doi: https://doi.org/10.1109/ACCESS.2018.2889437
S. Ismail, I. Siddiqi, U. Akram, “Localization and classification of heart beats in phonocardiography signals —a comprehensive review,” EURASIP J. Adv. Signal Process., vol. 2018, art. no. 26, 2018, doi: https://doi.org/10.1186/s13634-018-0545-9
W. Chen, Q. Sun, X. Chen, G. Xie, H. Wu, C. Xu, “Deep Learning Methods for Heart Sounds Classification: A Systematic Review,” Entropy, vol. 23, no. 6, art. no. 667, 2021, doi: https://doi.org/10.3390/e23060667
C. Liu, D. Springer, Q. Li, B. Moody, R. A. Juan, F. J. Chorro, F. Castells, J. M. Roig, I. Silva, A. E. W. Johnson, et al., “An open access database for the evaluation of heart sound algorithms,” Physiol. Meas., vol. 37, no. 12, art. no. 2181, Nov. 2016 doi: https://doi.org/10.1088/0967-3334/37/12/2181
S. G. Mallat, Z. Zhang, “Matching pursuits with time-frequency dictionaries,” IEEE Trans. Signal Process., vol. 41, no. 12, pp. 3397–3415, Dec. 1993, doi: https://doi.org/10.1109/78.258082
R. F. Ibarra, M. A. Alonso, S. Villarreal, C. I. Nieblas, “A parametric model for heart sounds,” in 2015 49th Asilomar Conference on Signals, Systems and Computers, Pacific Grove, CA, USA, 2015, pp. 765-769, doi: https://doi.org/10.1109/ACSSC.2015.7421237
C.I. Nieblas, M. A. Alonso, R. Conte, S. Villarreal, “High performance heart sound segmentation algorithm based on Matching Pursuit,” 2013 IEEE Digital Signal Processing and Signal Processing Education Meeting (DSP/SPE), Napa, CA, USA, 2013, pp. 96-100, doi: https://doi.org/10.1109/DSP-SPE.2013.6642572
W. Wang, Z. Guo, J. Yang, Y. Zhang, L.-G. Durand, M. Loew, “Analysis of the first heart sound using the matching pursuit method,” Med. Biol. Eng. Comput., vol. 39, pp. 644-648, Nov. 2001, doi: https://doi.org/10.1007/BF02345436
Xuan Zhang, L. Durand, L. Senhadji, H. C. Lee, J.-L. Coatrieux, “Analysis-synthesis of the phonocardiogram based on the matching pursuit method,” IEEE Trans. Biomed. Eng., vol. 45, no. 8, pp. 962-971, Aug. 1998, doi: https://doi.org/10.1109/10.704865
S. Jabbari, H. Ghassemian, “Modeling of heart systolic murmurs based on multivariate matching pursuit for diagnosis of valvular disorders,” Comput. Biol. Med., vol. 41, no. 9, pp. 802-811, Sep. 2011, doi: https://doi.org/10.1016/j.compbiomed.2011.06.016
W. Wang, J. Pan, H. Lian, “Decomposition and analysis of the second heart sound based on the matching pursuit method,” in Proceedings 7th International Conference on Signal Processing, 2004, Beijing, China, 2004, pp. 2229-2232, vol. 3, doi: https://doi.org/10.1109/ICOSP.2004.1442222
P. P. Vaidyanathan, “The Theory of Linear Prediction,” in Synthesis Lectures on Signal Processing. Switzerland: Springer Nature, 2022, pp. 1-184, doi: https://doi.org/10.2200/S00086ED1V01Y200712SPR003
T. Virtanen, M. D. Plumbley, D. Ellis, Computational analysis of sound scenes and events. Springer Cham, 2018, pp. 1-422, doi: https://doi.org/10.1007/978-3-319-63450-0
L. Breiman, “Random Forests,” Mach. Learn., vol. 45, pp. 5-32, 2001, doi: https://doi.org/10.1023/A:1010933404324
R. Gonzalez-Landaeta, B. Ramirez, J. Mejia, “Estimation of systolic blood pressure by Random Forest using heart sounds and a ballistocardiogram,” Sci. Rep., vol. 12, art. no. 17196, doi: https://doi.org/10.1038/s41598-022-22205-0
C. C. Balili, M. C. C. Sobrepena, P. C. Naval, “Classification of heart sounds using discrete and continuous wavelet transform and random forests,” in 2015 3rd IAPR Asian Conference on Pattern Recognition (ACPR), Kuala Lumpur, Malaysia, 2015, pp. 655-659, doi: https://doi.org/10.1109/ACPR.2015.7486584
W. Xu, K. Yu, J. Ye, H. Li, J. Chen, F. Yin, et al., “Automatic pediatric congenital heart disease classification based on heart sound signal,” Artif. Intell. Med., vol. 126, art. no. 102257, Apr. 2022, doi: https://doi.org/10.1016/j.artmed.2022.102257
J. Oliveira, D. Nogueira, C. Ferreira, A. M. Jorge, M. Coimbra, “The robustness of Random Forest and Support Vector Machine Algorithms to a Faulty Heart Sound Segmentation,” in 2022 44th Annual International Conference of the IEEE Engineering in Medicine & Biology Society (EMBC), Glasgow, Scotland, United Kingdom, 2022, pp. 1989-1992, doi: https://doi.org/10.1109/EMBC48229.2022.9871111
M. Y. Esmail, D. H. Ahmed, M. Eltayeb, “Classification System for Heart Sounds Based on Random Forests,” J. Clin. Eng., vol. 44, no. 2, pp. 76-80, 2019, doi: https://doi.org/10.1097/JCE.0000000000000335
N. V. Chawla, K. W. Bowyer, L. O. Hall, W. P. Kegelmeyer, “Smote: Synthetic Minority Over-sampling Technique,” J. Artif. Intell. Res., vol. 16, pp. 321–357, Jun. 2002, doi: https://doi.org/10.1613/jair.953
R. F. Ibarra-Hernández, M. A. Alonso-Arévalo, A. Cruz-Gutiérrez, A. L. Licona-Chávez, S. Villarreal-Reyes, “Design and evaluation of a parametric model for cardiac sounds,” Comput. Biol. Med., vol. 89, pp. 170–180, Oct. 2017, doi: https://doi.org/10.1016/j.compbiomed.2017.08.007
L. L. Vercio, M. Del Fresno, I. Larrabide, “Detection of morphological structures for vessel wall segmentation in ivus using random forests,” in 12th International Symposium on Medical Information Processing and Analysis, Tandil, Argentina, 2017, art. no. 1016012, doi: https://doi.org/10.1117/12.2255748
A. Mellor, S. Boukir, A. Haywood, S. Jones, “Exploring issues of training data imbalance and mislabelling on random forest performance for large area land cover classification using the ensemble margin,” ISPRS J. Photogramm. Remote Sens., vol. 105, pp. 155–168, Jul. 2015, doi: https://doi.org/10.1016/j.isprsjprs.2015.03.014
R. F. Ibarra-Hernández, N. Bertin, M. A. Alonso-Arévalo, H. A. Guillén-Ramírez, “A benchmark of heart sound classification systems based on sparse decompositions,” in 14th International Symposium on Medical Information Processing and Analysis, Maztlán, México, 2018, art. no. 1097505, doi: https://doi.org/10.1117/12.2506758
F. Pedregosa, G. Varoquaux, A. Gramfort, V. Michel, B. Thirion, O. Grisel, et al., “Scikit-learn: Machine Learning in Python,” J. Mach. Learn. Res., vol. 12, pp. 2825–2830, 2011. [Online] Available: https://www.jmlr.org/papers/volume12/pedregosa11a/pedregosa11a.pdf?ref=https:/
M. A. Hall, “Correlation-based feature selection for machine learning,” PhD dissertation, University of Waikato, Hamilton, New Zeland, 1999. [Online]. Available: https://hdl.handle.net/10289/15043
B. Azhagusundari, A. S. Thanamani, “Feature selection based on information gain,” IJITEE, vol. 2, no. 2, pp. 18-21, Jan. 2013. [Online]. Available: https://citeseerx.ist.psu.edu/document?repid=rep1&type=pdf&doi=e17df473c25cccd8435839c9b6150ee61bec146a
S. Krstulovic, R. Gribonval, “Mptk: Matching Pursuit Made Tractable,” 2006 IEEE International Conference on Acoustics Speech and Signal Processing Proceedings, Toulouse, Francia, 2006, pp. III-III, doi: https://doi.org/10.1109/ICASSP.2006.1660699
G. D. Clifford, C. Liu, B. Moody, J. Millet, S. Schmidt, Q. Li, et al., “Recent advances in heart sound analysis,” Physiol. Meas., vol. 38, no. 8, art. no. E10, Aug. 2017, doi: https://doi.org/10.1088/1361-6579/aa7ec8
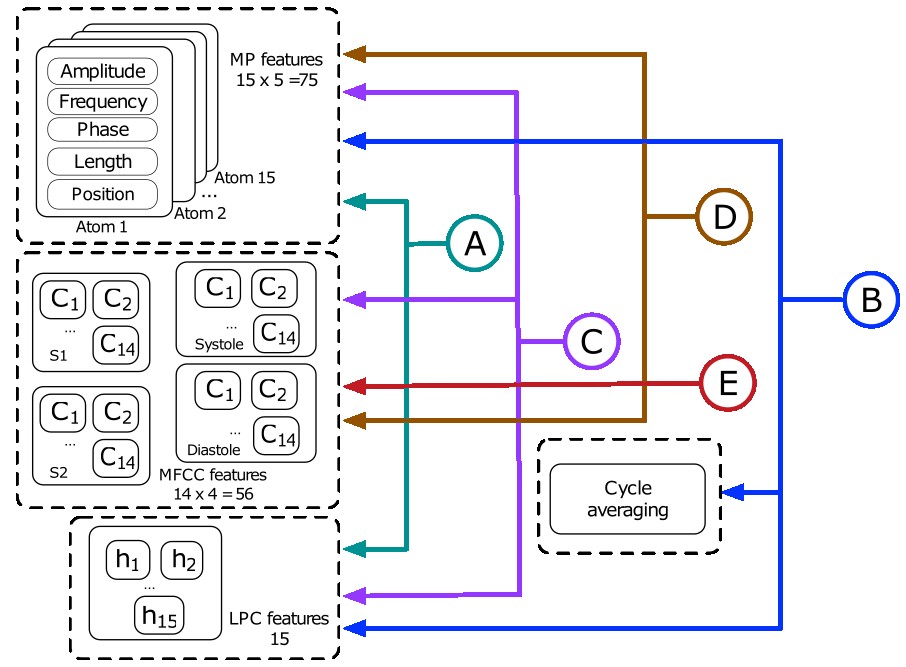
Downloads
Published
How to Cite
Issue
Section
License
Copyright (c) 2023 Revista Mexicana de Ingenieria Biomedica

This work is licensed under a Creative Commons Attribution-NonCommercial 4.0 International License.
Upon acceptance of an article in the RMIB, corresponding authors will be asked to fulfill and sign the copyright and the journal publishing agreement, which will allow the RMIB authorization to publish this document in any media without limitations and without any cost. Authors may reuse parts of the paper in other documents and reproduce part or all of it for their personal use as long as a bibliographic reference is made to the RMIB. However written permission of the Publisher is required for resale or distribution outside the corresponding author institution and for all other derivative works, including compilations and translations.