Evaluación de la Calidad de los Agentes Conversacionales para la Creación de Instrumentos de Evaluación en Medición de Señales Bioeléctricas
DOI:
https://doi.org/10.17488/RMIB.44.4.11Palabras clave:
Bard, Chat-GPT, evaluación educativa, ingeniería biomédica, inteligencia artificialResumen
El objetivo de esta investigación es evaluar la calidad de agentes conversacionales basados en Modelos de Lenguaje Grandes, para la evaluación de aplicación de conocimiento en Ingeniería Biomédica. Se desarrolló un instrumento de evaluación sobre seis temas de medición de señales bioeléctricas elaborado por un agente humano y los agentes conversacionales Chat-GPT y Bard. Se evaluó la calidad del instrumento en términos de nivel de pensamiento, validez, relevancia, claridad, dificultad y capacidad de discriminación, mediante índice kappa (k) del acuerdo de dos expertos y análisis Rasch de resultados de treinta y ocho estudiantes. Tras eliminar siete preguntas de los agentes conversacionales por problemas de validez y originalidad se integró un instrumento de seis preguntas. Las preguntas fueron válidas y relevantes, claras (>0.95, k=1.0), con dificultad baja a alta (0.61-0.87, k=0.83), índice de discriminación adecuado (0.11-0.47), a nivel de pensamiento de análisis (k=0.22). El promedio de los estudiantes fue de 7.24±2.40. Este es el primer análisis crítico de la calidad de los agentes conversacionales a un nivel de pensamiento superior al de comprensión. Los agentes conversacionales presentaron limitaciones en términos de validez, originalidad, dificultad y discriminación en comparación con el experto humano lo que resalta la necesidad aún de su supervisión.
Descargas
Citas
OpenAI, “ChatGPT” [Large language model]. OpenAI. https://chat.openai.com/chat (consultado en 2023).
Google, “Bard” [Large Language Model]. 2023. https://bard.google.com/chat (consultado en 2023).
M. Sallam, “ChatGPT Utility in Healthcare Education, Research, and Practice: Systematic Review on the Promising Perspectives and Valid Concerns,” Healthcare, vol. 11, no. 6, art. no. 887, mar. 2023, doi: https://doi.org/10.3390/healthcare11060887
M. McTear, Z. Callejas, D. Griol, “The conversational interface: Talking to smart devices,” in The Conversational Interface, Switzerland: Springer International Publishing, 2016, doi: https://doi.org/10.1007/978-3-319-32967-3
M. M. E. Van Pinxteren, M. Pluymaekers, J. G. A. M. Lemmink, “Human-like communication in conversational agents: a literature review and research agenda,” J. Serv. Manag., vol. 31, no. 2, pp. 203–225, 2020, doi: https://doi.org/10.1108/JOSM-06-2019-0175
J. Manyika, “An overview of Bard: an early experiment with generative AI,” Google. 2023. [En línea]. Disponible en: https://ai.google/static/documents/google-about-bard.pdf
T. B. Brown, B. Mann, N. Ryder, M. Subbiah, et al., “Language models are few-shot learners,” 2020, arXiv:2005.14165, doi: https://doi.org/10.48550/arXiv.2005.14165
O. Vinyals, Q. Le, “A Neural Conversational Model,” 2015, arXiv: 1506.05869, doi : https://doi.org/10.48550/arXiv.1506.05869
T. Bianchi, “Global search volume for ‘ChatGPT API’, ‘AI API’ keywords 2022-2023,” Statista. Disponible en: https://www.statista.com/statistics/1398265/chatgpt-ai-api-keywords-search-volume/ (consultado el 26 de octubre de 2023).
A. Petrosyan, “ChatGPT and cyber crime - Statistics & Facts,” Statista. Disponible en: https://www.statista.com/topics/10818/chatgpt-and-cyber-crime/#topicOverview (consultado el 26 de octubre de 2023).
R. S. D'Amico, T. G. White, H. A. Shah, D. J. Langer, “I Asked a ChatGPT to Write an Editorial About How We Can Incorporate Chatbots Into Neurosurgical Research and Patient Care…,” Neurosurgery, vol. 92, no. 4, pp. 663–664, abr. 2023, doi: https://doi.org/10.1227/neu.0000000000002414
F. Y. Al-Ashwal, M. Zawiah, L. Gharaibeh, R. Abu-Farha, A. N. Bitar, “Evaluating the Sensitivity, Specificity, and Accuracy of ChatGPT-3.5, ChatGPT-4, Bing AI, and Bard Against Conventional Drug-Drug Interactions Clinical Tools,” Drug Healthc. Patient. Saf., vol. 15, pp. 137–147, sep. 2023, doi: https://doi.org/10.2147/dhps.s425858
H. Yang, “How I use ChatGPT responsibly in my teaching,” Nature, apr. 2023, doi: https://doi.org/10.1038/d41586-023-01026-9
S. Ariyaratne, K. P. Iyengar, N. Nischal, N. Chitti Babu, R. Botchu, “A comparison of ChatGPT-generated articles with human-written articles,” Skeletal Radiol., vol. 52, no. 9, pp. 1755–1758, sep. 2023, doi: https://doi.org/10.1007/s00256-023-04340-5
G. Eysenbach, “The Role of ChatGPT, Generative Language Models, and Artificial Intelligence in Medical Education: A Conversation With ChatGPT and a Call for Papers,” JMIR Med. Educ., vol. 9, no. 4, art. no. e46885, mar. 2023, doi: https://doi.org/10.2196/46885
D. De Silva, N. Mills, M. El-Ayoubi, M. Manic, D. Alahakoon, “ChatGPT and Generative AI Guidelines for Addressing Academic Integrity and Augmenting Pre-Existing Chatbots,” in 2023 IEEE International Conference on Industrial Technology (ICIT), Orlando, FL, USA, 2023, pp. 1–6. doi: https://doi.org/10.1109/ICIT58465.2023.10143123
M. J. Bommarito, D. M. Katz, “GPT Takes the Bar Exam,” SSRN Electron. J., pp. 1–13, 2023, doi: https://dx.doi.org/10.2139/ssrn.4314839
J. Bommarito, M. J. Bommarito, J. Katz, D. M. Katz, “Gpt as Knowledge Worker: A Zero-Shot Evaluation of (AI)CPA Capabilities,” SSRN Electron. J., 2023, doi: https://dx.doi.org/10.2139/ssrn.4322372
A. Gilson, C. W. Safranek, T. Huang, V. Socrates, L. Chi, R. A. Taylor, D. Chartash, “How Does ChatGPT Perform on the United States Medical Licensing Examination? The Implications of Large Language Models for Medical Education and Knowledge Assessment,” JMIR Med. Educ., vol. 9, art. no. e45312, 2023, doi: https://doi.org/10.2196/45312
T. H. Kung, M. Cheathem, A. Medenilla, C. Sillos, et al., “Performance of ChatGPT on USMLE: Potential for AI-assisted medical education using large language models,” PLOS Digit. Health, vol. 2, no. 2, art. no. e0000198, 2023, doi: https://doi.org/10.1371/journal.pdig.0000198
J. Miao, C. Thongprayoon, W. Cheungpasitporn, “Assessing the Accuracy of ChatGPT on Core Questions in Glomerular Disease,” Kidney Int. Rep., vol. 8, no. 8, pp. 1657–1659, 2023, doi: https://doi.org/10.1016/j.ekir.2023.05.014
B. H. H. Cheung, G. K. K. Lau, G. T. C. Wong, E. Y. P. Lee, et al., “ChatGPT versus human in generating medical graduate exam multiple choice questions—A multinational prospective study (Hong Kong S.A.R., Singapore, Ireland, and the United Kingdom),” PLoS One, vol. 18, no. 8, art. no. e0290691, ago. 2023, doi: https://doi.org/10.1371/journal.pone.0290691
A. K. Khilnani, “Potential of Large Language Model (ChatGPT) in Constructing Multiple Choice Questions,” GAIMS J. Med. Sci., vol. 3, no. 2, pp. 1–3, 2023. [En línea]. Disponible en: https://gjms.gaims.ac.in/index.php/gjms/article/view/71
S. Elkins, E. Kochmar, I. Serban, J. C. K. Cheung, “How Useful Are Educational Questions Generated by Large Language Models?,” in Artificial Intelligence in Education. Posters and Late Breaking Results, Workshops and Tutorials, Industry and Innovation Tracks, Practitioners, Doctoral Consortium and Blue Sky. AIED 2023. Communications in Computer and Information Science, vol 1831, Tokyo, Japón, 2023, pp. 536–542, doi: https://doi.org/10.1007/978-3-031-36336-8_83
X. Yuan, T. Wang, Y.-H. Wang, E. Fine, R. Abdelghani, H. Sauzéon, P.-Y. Oudeyer, “Selecting Better Samples from Pre-trained LLMs: A Case Study on Question Generation,” in Findings of the Association for Computational Linguistics: ACL 2023, Toronto, Canadá, 2023, pp. 12952–12965, doi: https://doi.org/10.18653/v1/2023.findings-acl.820
C. Xiao, S. X. Xu, K. Zhang, Y. Wang, L. Xia, “Evaluating Reading Comprehension Exercises Generated by LLMs: A Showcase of ChatGPT in Education Applications,” in Proceedings of the 18th Workshop on Innovative Use of NLP for Building Educational Applications (BEA 2023), Toronto, Canadá, 2023, pp. 610–625, doi: https://doi.org/10.18653/v1/2023.bea-1.52
S. Sedaghat, “Early applications of ChatGPT in medical practice, education and research,” Clin. Med., vol. 23, no. 3, pp. 278–279, may. 2023, doi: https://doi.org/10.7861/clinmed.2023-0078
R. Dijkstra, Z. Genç, S. Kayal, and J. Kamps, “Reading Comprehension Quiz Generation using Generative Pre-trained Transformers,” in 4th International Workshop on Intelligent Textbooks, iTextbooks 2022, Durham, Reino Unido, 2022. [En línea]. Disponible en: https://hdl.handle.net/11245.1/a1109043-92d4-4c63-be33-6e238780d3b7
L. W. Anderson, D. R. Krathwohl, P. W. Airasian, K. A. Cruikshank, et al., A Taxonomy for Learning, Teaching, and Assessing: A Revision of Bloom’s Taxonomy of Educational Objectives. Nueva York: Pearson Education, 2001.
Jamovi. (2023). [En línea]. Disponible en: https://www.jamovi.org
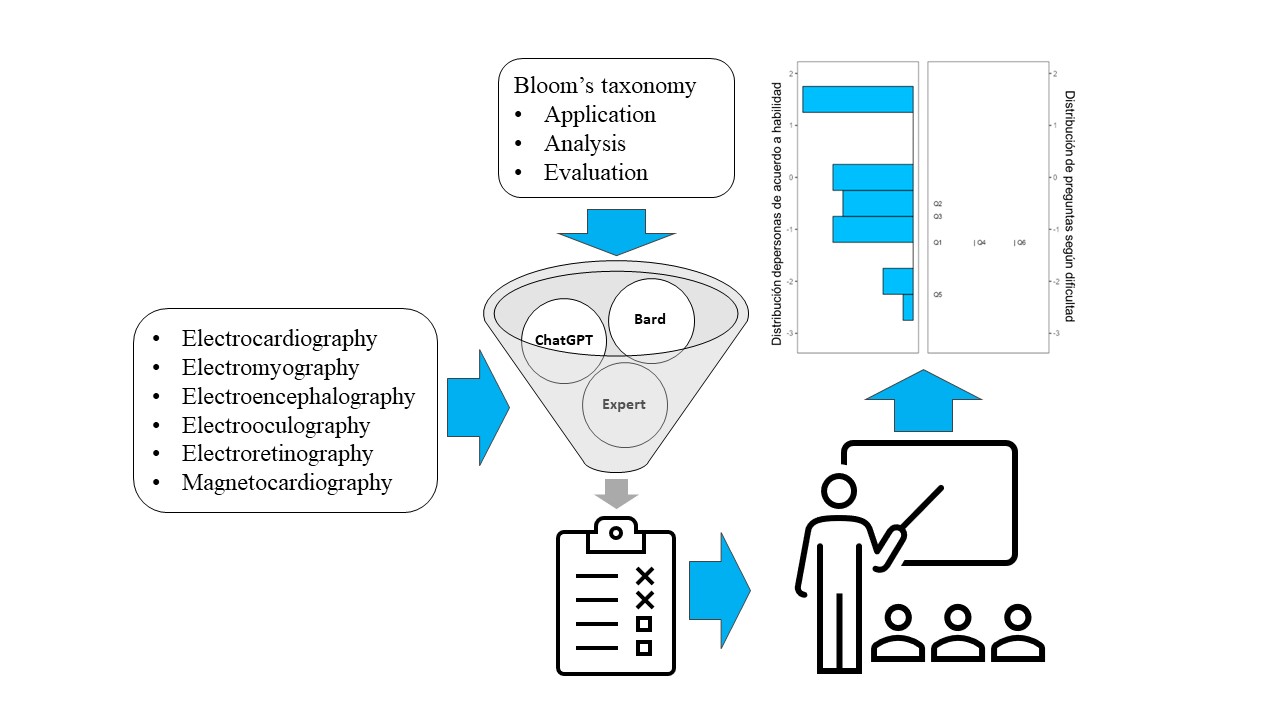
Publicado
Cómo citar
Número
Sección
Licencia
Derechos de autor 2023 Revista Mexicana de Ingenieria Biomedica

Esta obra está bajo una licencia internacional Creative Commons Atribución-NoComercial 4.0.
Una vez que el artículo es aceptado para su publicación en la RMIB, se les solicitará al autor principal o de correspondencia que revisen y firman las cartas de cesión de derechos correspondientes para llevar a cabo la autorización para la publicación del artículo. En dicho documento se autoriza a la RMIB a publicar, en cualquier medio sin limitaciones y sin ningún costo. Los autores pueden reutilizar partes del artículo en otros documentos y reproducir parte o la totalidad para su uso personal siempre que se haga referencia bibliográfica al RMIB. No obstante, todo tipo de publicación fuera de las publicaciones académicas del autor correspondiente o para otro tipo de trabajos derivados y publicados necesitaran de un permiso escrito de la RMIB.