Estudio de la Longitud de Ventana de Tiempo en el Reconocimiento de Emociones Basado en Señales EEG
DOI:
https://doi.org/10.17488/RMIB.45.1.3Palabras clave:
aprendizaje automático, electroencefalograma, longitud de ventana de tiempo, reconocimiento de emocionesResumen
El objetivo de esta investigación es presentar un análisis comparativo empleando diversas longitudes de ventanas de tiempo (VT) durante el reconocimiento de emociones, utilizando técnicas de aprendizaje automático y el dispositivo de sensado inalámbrico portátil EPOC+. En este estudio, se utilizará la entropía como característica para evaluar el rendimiento de diferentes modelos clasificadores en diferentes longitudes de VT, basándose en un conjunto de datos de señales EEG extraídas de individuos durante la estimulación de emociones. Se llevaron a cabo dos tipos de análisis: entre sujetos e intra-sujetos. Se compararon las medidas de rendimiento, tales como la exactitud, el área bajo la curva y el coeficiente de Cohen's Kappa, de cinco modelos clasificadores supervisados: K-Nearest Neighbors (KNN), Support Vector Machine (SVM), Logistic Regression (LR), Random Forest (RF) y Decision Trees (DT). Los resultados indican que, en ambos análisis, los cinco modelos presentan un mayor rendimiento en VT de 2 a 15 segundos, destacándose especialmente la VT de 10 segundos para el análisis entre los sujetos y 5 segundos intra-sujetos; además, no se recomienda utilizar VT superiores a 20 segundos. Estos hallazgos ofrecen una orientación valiosa para la elección de las VT en el análisis de señales EEG al estudiar las emociones.
Descargas
Citas
P. Salovey and J. D. Mayer, “Emotional intelligence. Imagination, cognition and personality,” Imagin. Cogn. Pers., vol. 9, no. 3, pp. 185-211, 1990, doi: https://psycnet.apa.org/doi/10.2190/DUGG-P24E-52WK-6CDG
R. W. Picard, “Affective Computing for HCI”, in 8th International Conference on Human-Computer Interaction) on Human-Computer Interaction: Ergonomics and User Interfaces-Volume I - Volume I, 1999, pp. 829-833, doi: https://dl.acm.org/doi/abs/10.5555/647943.742338
R. Stock-Homburg, “Survey of emotions in human–robot interactions: Perspectives from robotic psychology on 20 years of research,” Int. J. Soc. Robotics, vol. 14, no. 2, pp. 389-411, Mar. 2022, doi: https://doi.org/10.1007/s12369-021-00778-6
M. S. Young, K. A. Brookhuis, C. D. Wickens and P. A. Hancock, “State of science: Mental workload in ergonomics,” Ergonomics, vol. 58, no. 1, Dec. 2014, doi: https://doi.org/10.1080/00140139.2014.956151
J. X. Chen, P. W. Zhang, Z. J. Mao, Y. F. Huang, D. M. Jiang, and Y. N. Zhang, “Accurate EEG-Based Emotion Recognition on Combined Features Using Deep Convolutional Neural Networks,” IEEE Access, vol. 7, pp. 44317-44328, Jun. 2019, doi: https://doi.org/10.1109/ACCESS.2019.2908285
C. Qing, R. Qiao, X. Xu, and Y. Cheng, “Interpretable Emotion Recognition Using EEG Signals,” IEEE Access, vol. 7, pp. 94160-94170, Jul. 2019, doi: https://doi.org/10.1109/ACCESS.2019.2928691
M. M. Duville, Y. Pérez, R. Hugues-Gudiño, N. E. Naal-Ruiz, L. M. Alonso-Valerdi, D. I. Ibarra-Zarate, “Systematic Review: Emotion Recognition Based on Electrophysiological Patterns for Emotion Regulation Detection,” Appl. Sci., vol. 13, no. 12, art. no. 6896, Feb. 2023, doi: https://doi.org/10.3390/app13126896
C. Pan, C. Shi, H. Mu, J. Li, and X. Gao, “EEG-Based Emotion Recognition Using Logistic Regression with Gaussian Kernel and Laplacian Prior and Investigation of Critical Frequency Bands,” Appl. Sci., vol. 9, no. 2, art. no. 1619, Apr. 2019, doi: https://doi.org/10.3390/app10051619
D. Wu, “Online and Offline Domain Adaptation for Reducing BCI Calibration Effort,” IEEE Trans. Hum.-Mach. Syst., vol. 47, no. 4, pp. 550-563, Aug. 2017, doi: https://doi.org/10.1109/THMS.2016.2608931
G. Li, D. Ouyang, Y. Yuan, W. Li, Z. Guo, X. Qu, P. Green, “An EEG Data Processing Approach for Emotion Recognition,” IEEE Sens. J., vol. 22, no. 11, pp. 10751-10763, Jun. 2022, doi: https://doi.org/10.1109/JSEN.2022.3168572
P. Schmidt, A. Reiss, R. Dürichen, and K. Van Laerhoven, “Wearable-Based Affect Recognition—A Review,” Sensors, vol. 19, no. 19, art. no. 4079, Sep. 2019, doi: https://doi.org/10.3390/s19194079
J. J. Esqueda Elizondo, L. Jiménez Beristáin, A. Serrano Trujillo, M. Zavala Arce, et al., “Using Machine Learning Algorithms on Electroencephalographic Signals to Assess Engineering Students' Focus While Solving Math Exercises,” Rev. Mex. Ing. Biom., vol. 44, no. 4, pp. 23-37, Nov. 2023, doi: https://doi.org/10.17488/RMIB.44.4.2
F. J. Ramírez-Arias, E. E. García-Guerrero, E. Tlelo-Cuautle, et al., “Evaluation of machine learning algorithms for classification of EEG signals,” Technologies, vol. 10, no 4, art. no. 79, Jun. 2022, doi: https://doi.org/10.3390/technologies10040079
M. Zheng and Y. Lin, “A deep transfer learning network with two classifiers based on sample selection for motor imagery brain-computer interface,” Biomed. Signal Process. Control, vol. 89, art. no. 105786, Mar. 2024, doi: https://doi.org/10.1016/j.bspc.2023.105786
J. J. Esqueda-Elizondo, R. Juárez-Ramírez, O. R. López-Bonilla, E. E. García-Guerrero, et al., “Attention measurement of an autism spectrum disorder user using EEG signals: A case study,” Math. Comput. Appl., vol. 27, no. 2, art. no. 21, Mar. 2022, doi: https://doi.org/10.3390/mca27020021
Y. Qin, B. Li, W. Wang, X. Shi, H. Wang, and X. Wang, “ETCNet: An EEG-based motor imagery classification model combining efficient channel attention and temporal convolutional network,” Brain Res., vol. 1823, art. no. 148673, Nov. 2023, doi: https://doi.org/10.1016/j.brainres.2023.148673
J. Li, S. Qiu, C. Du, Y. Wang, and H. He, “Domain Adaptation for EEG Emotion Recognition Based on Latent Representation Similarity,” IEEE Trans, Cogn. Dev. Syst., vol. 12, no. 2, pp. 344-353, Jun. 2020, doi: https://doi.org/10.1109/TCDS.2019.2949306
Y. -P. Lin, C.-H. Wang, T.P. Jung, T.-L. Wu, S.-K. Jeng, J.-R. Duann, J.-H. Chen, “EEG-Based Emotion Recognition in Music Listening”, IEEE Trans. Biomed. Eng., vol. 57, no. 7, pp. 1798-1806 Jul. 2010, doi: https://doi.org/10.1109/tbme.2010.2048568
W.-L. Zheng, B.-N. Dong, and B.-L. Lu, “Multimodal emotion recognition using EEG and eye tracking data,” in 2014 36th Annual International Conference of the IEEE Engineering in Medicine and Biology Society, Chicago, IL, USA, 2014, pp. 5040-5043, doi: https://doi.org/10.1109/EMBC.2014.6944757
N. Zhuang, Y. Zeng, L. Tong, C. Zhang, H. Zhang, and B. Yan, “Emotion recognition from EEG signals using multidimensional information in EMD domain,” Biomed Res. Int., vol. 2017, art. no. 8317357, 2017, doi: https://doi.org/10.1155/2017/8317357
D. Ouyang, Y. Yuan, G. Li, and Z. Guo, “The Effect of Time Window Length on EEG-Based Emotion Recognition,” Sensors, vol. 22, no. 13, art. no. 4939, Jun. 2022, doi: https://doi.org/10.3390/s22134939
J. Healey, L. Nachman, S. Subramanian, J. Shahabdeen, and M. Morris, “Out of the Lab and into the Fray: Towards Modeling Emotion in Everyday Life,” in 8th International Conference, Pervasive 2010, P. Floréen, A. Krüger, M. Spasojevic, Eds., Helsinki, Finland 2010, doi: https://doi.org/10.1007/978-3-642-12654-3_10
M. Gjoreski, M. Luštrek, M. Gams, and H. Gjoreski, “Monitoring stress with a wrist device using context,” J. Biomed. Inform., vol. 73, pp. 159-170, Sep. 2017, doi: https://doi.org/10.1016/j.jbi.2017.08.006
R. Alhalaseh and S. Alasasfeh, “Machine-Learning-Based Emotion Recognition System Using EEG Signals,” Computers, vol 9, no 4, art. no. 95, 2020, doi: https://doi.org/10.3390/computers9040095
K. S. Kamble and J. Sengupta, “Ensemble Machine Learning-Based Affective Computing for Emotion Recognition Using Dual-Decomposed EEG Signals,” IEEE Sens. J., vol. 22, no. 3, pp. 2496-2507, Feb. 2022, doi: https://doi.org/10.1109/JSEN.2021.3135953
X. Li, D. Song, P. Zhang, Y. Zhang, Y. Hou, and B. Hu, “Exploring EEG Features in Cross-Subject Emotion Recognition,” Front. Neurosci., vol 12, art. no. 162, Mar. 2018, doi: https://doi.or/10.3389/fnins.2018.00162
B. Tripathi and R. K. Sharma, “EEG-Based Emotion Classification in Financial Trading Using Deep Learning: Effects of Risk Control Measures,” Sensors, vol. 23, no. 7, art. no. 3474, Mar. 2023, doi: https://doi.org/10.3390/s23073474
M. D. Rinderknecht, O. Lambercy, and R. Gassert, “Enhancing simulations with intra-subject variability for improved psychophysical assessments,” PLoS One, vol. 13, no. 12, art. no. e0209839, Dec. 2018, doi: https://doi.org/10.1371/journal.pone.0209839
A. Jarillo-Silva, V. A. Gomez-Perez, E. A Escotto-Cordova, and O. A. Domínguez-Ramírez, “Emotion Classification form EEG signals using wearable sensors:pilot test,” ECORFAN Journal-Bolivia, vol. 7, no. 12, pp. 1-9, Sep. 2020. [Online]. Available: https://www.ecorfan.org/bolivia/journal/vol7num12/ECORFAN_Journal_Bolivia_V7_N12.pdf
K. Kotowski, K. Stapo, J. Leski, and M. Kotas, “Validation of Emotiv EPOC+ for extracting ERP correlates of emotional face processing,” Biocybern. Biomed. Eng., vol. 38, no 4, pp. 773-781, 2018, doi: https://doi.org/10.1016/j.bbe.2018.06.006
F. Mulla, E. Eya, E. Ibrahim, A. Alhaddad, R. Qahwaji, and R. Abd-Alhameed, “Neurological assessment of music therapy on the brain using Emotiv Epoc,” in 2017 Internet Technologies and Applications (ITA), Wrexham, UK, 2017, pp. 259-263, doi: https://doi.org/10.1109/ITECHA.2017.8101950
N. Browarska, A. Kawala-Sterniuk, J. Zygarlicki, M. Podpora, M. Pelc, R. Martinek, and E. J. Gorzelańczyk, “Comparison of smoothing filters influence on quality of data recorded with the emotiv epoc flex brain–computer interface headset during audio stimulation,” Brain Sci., vol. 11, no. 1, art. no. 98, Jan. 2021, doi: https://doi.org/10.3390/brainsci11010098
P. R. Patel and R. N. Annavarapu, “EEG-based human emotion recognition using entropy as a feature extraction measure,” Brain Inf., vol 8, art. no. 20, Oct. 2021, doi: https://doi.org/10.1186/s40708-021-00141-5
P. Krishnan and S. Yaacob, “Drowsiness detection using band power and log energy entropy features based on EEG signals,” Int. J. Innov. Technol. Explor. Eng, vol 8, no. 10, pp. 830-836, Aug. 2019, doi: https://doi.org/10.35940/ijitee.j9025.0881019
A. B Das and M. I. H. Bhuiyan, “Discrimination and classification of focal and non-focal EEG signals using entropy-based features in the EMD-DWT domain,” Biomed. Signal Process. Control, vol. 29, pp. 11-21, Aug. 2016, doi: https://doi.org/10.1016/j.bspc.2016.05.004
R. Djemal, K. AlSharabi, S. Ibrahim, and A. Alsuwailem, “EEG-Based Computer Aided Diagnosis of Autism Spectrum Disorder Using Wavelet, Entropy, and ANN,” BioMed Res. Int., vol. 2017, no 1, art. no. 9816591, 2017, doi: https://doi.org/10.1155/2017/9816591
S. Koelstra, C. Muhl, M. Soleymani, J.-S. Lee, et al., “DEAP: A Database for Emotion Analysis; Using Physiological Signals,” IEEE Trans. Affect. Comput., vol. 3, no. 1, pp. 18-31, 2012, doi: https://doi.org/10.1109/T-AFFC.2011.15
A. Bablani, D. Reddy Edla, and S. Dodia, “Classification of EEG Data using k-Nearest Neighbor approach for Concealed Information Test,” Procedia Comput. Sci., vol. 143, pp. 242-249, 2018, doi: https://doi.org/10.1016/j.procs.2018.10.392
D.-W. Chen, R. Miao, W.-Q. Yang, Y. Liang, H.-H. Chen, L. Huang, C.-J. Deng, N. Han, “A feature extraction method based on differential entropy and linear discriminant analysis for emotion recognition,” Sensors, vol. 19, no. 7, art. no. 1631, Oct. 2019, doi: https://doi.org/10.3390/s19071631
S. Babeetha and S. S. Sridhar, “EEG Signal Feature Extraction Using Principal Component Analysis and Power Spectral Entropy for Multiclass Emotion Prediction,” in Fourth International Conference on Image Processing and Capsule Networks, Bangkok, Thailand, 2023, pp. 435-448, doi: https://doi.org/10.1007/978-981-99-7093-3_29
V. Doma and M. Pirouz, “A comparative analysis of machine learning methods for emotion recognition using EEG and peripheral physiological signals,” J. Big Data, vol. 7, art. no. 18, Mar. 2020, doi: https://doi.org/10.1186/s40537-020-00289-7
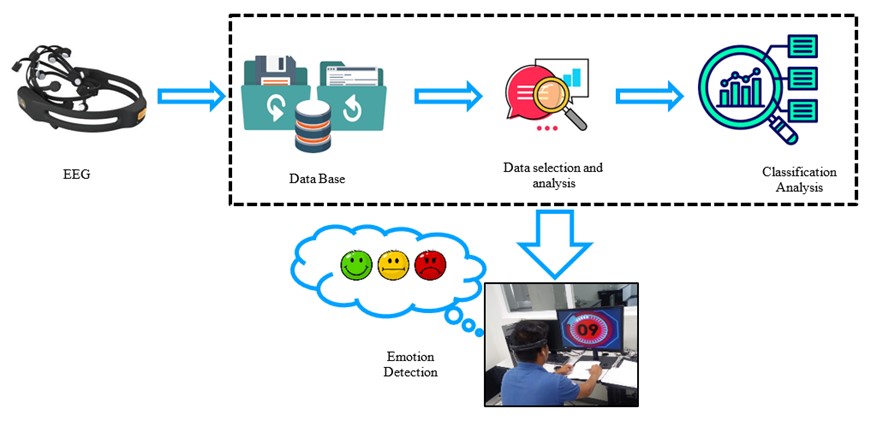
Descargas
Publicado
Cómo citar
Número
Sección
Licencia
Derechos de autor 2024 Revista Mexicana de Ingenieria Biomedica

Esta obra está bajo una licencia internacional Creative Commons Atribución-NoComercial 4.0.
Una vez que el artículo es aceptado para su publicación en la RMIB, se les solicitará al autor principal o de correspondencia que revisen y firman las cartas de cesión de derechos correspondientes para llevar a cabo la autorización para la publicación del artículo. En dicho documento se autoriza a la RMIB a publicar, en cualquier medio sin limitaciones y sin ningún costo. Los autores pueden reutilizar partes del artículo en otros documentos y reproducir parte o la totalidad para su uso personal siempre que se haga referencia bibliográfica al RMIB. No obstante, todo tipo de publicación fuera de las publicaciones académicas del autor correspondiente o para otro tipo de trabajos derivados y publicados necesitaran de un permiso escrito de la RMIB.